How Image Annotation Enhances Medical Diagnosis and Treatment

The integration of machine learning and artificial intelligence has propelled significant advancements in healthcare, particularly through the enhancement of image analysis. At the heart of these advancements lies image and data annotation services, crucial for creating annotated datasets that train machine learning models revolutionizing healthcare diagnostics, treatment planning, and disease monitoring.
By labeling and categorizing medical images from radiology, histology, and pathology, image annotation enables precise and efficient analysis. This process is vital for identifying critical areas such as tumors, lesions, and anatomical structures, significantly speeding up the detection and diagnosis by leveraging machine learning algorithms.
Furthermore, medical image annotation is indispensable in bridging the gap between raw medical images—captured via X-rays, MRIs, CT scans, and ultrasounds—and actionable insights. By marking specific areas within these images, healthcare professionals gain access to vital information, enhancing diagnostic accuracy, guiding surgical procedures, and fostering research.
This capability is pivotal not only for radiologists, who can detect abnormalities early, but also for surgeons, who rely on annotated images for surgical precision, and researchers, who utilize these images to explore disease progression and treatment responses. Consequently, data and image annotation services are fundamental to improving patient outcomes and accelerating medical innovations, underscoring their importance in the healthcare industry's technological evolution.

Healthcare Data Annotation Tools Market size was valued at USD 167.40 Million in 2023 and is projected to reach USD 719.15 Million by 2030, growing at a CAGR of 27.5% from 2024-2030.
Exploring Medical Image Annotation: Types, Tools, and Ethical Considerations
Types of Medical Image Annotation
Image Classification
This essential method categorizes medical images to help quickly identify and understand their key features, enhancing decision-making.
- Pathology Identification: Helps pinpoint diseases like tumors or fractures within images, guiding treatment strategies.
- Disease Severity Categorization: Useful for assessing the progression of conditions, aiding in determining appropriate medical interventions.
Object Detection
Focuses on accurately locating and delineating anomalies or structures, which is crucial for diagnosing and planning treatments.
- Anomaly Localization: Aids in identifying conditions early by highlighting critical areas needing examination.
- Organ and Structure Detection: Facilitates surgical planning by accurately marking organs and other structures.
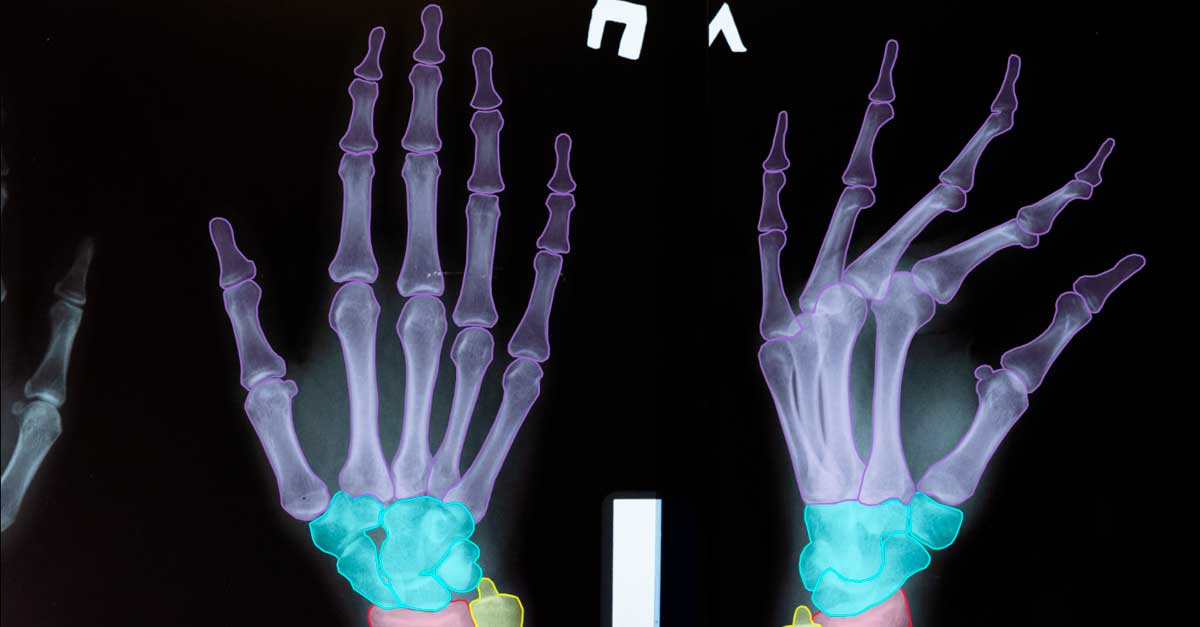
Semantic Segmentation
Offers pixel-level detail in mapping tissue types, which is crucial for detailed analysis and treatment planning.
- Pixel-Level Detailing: Assigns labels to every pixel, creating a comprehensive tissue map.
- Tissue Type Mapping: Enables detailed tissue distribution study, critical for identifying disease impact.
Landmark Annotation
Targets specific points within an image to assist in accurate measurements and analyses, crucial for surgical planning and post-operative evaluations.
- Precise Measurements: Identifies anatomical landmarks for detailed studies and surgical accuracy.
- Surgical Guidance: Helps in planning and evaluating surgeries through detailed landmark mapping.
3D Image Annotation
Essential for understanding complex structures in three-dimensional space, improving diagnostics and surgical planning.
- Volumetric Data Analysis: Enhances the visualization of anatomical relationships through detailed 3D annotations.
- Radiology and Surgery Applications: Aids in identifying abnormalities and planning surgeries with 3D models.
Tools and Technologies for Medical Image Annotation
Manual Annotation
Relies on the expertise of medical professionals to ensure accuracy, which is particularly vital for complex images.
- Expert Annotations: Utilizes the specialized knowledge of medical experts for precise labeling.
- Crowdsourced Annotations: Leverages a distributed workforce for large datasets, requiring quality control for accuracy.
Semi-Automated Annotation
Blends human expertise with automation to improve efficiency without sacrificing accuracy.
- Active Learning: Focuses annotation efforts on complex cases to enhance model performance with minimal human input.
- Pretrained Model Adaptation: Utilizes existing models for faster, more specific medical image annotations.
Annotation Software
Provides specialized tools for medical image annotation, making the process more efficient and user-friendly.
- Specialized Tools: Designed for medical needs, offering features like pixel-level segmentation and 3D annotations.
- General Tools: Offers versatility for simpler tasks, not specifically designed for medical images but still useful.
Challenges in Medical Image Annotation
Despite its benefits, annotation faces challenges like annotator variability, data privacy concerns, large dataset management, lack of standardization, and balancing speed with accuracy. Addressing these requires technological advances, standardized protocols, and quality control.
Best Practices for Accurate Medical Image Annotation
To maintain accuracy, it's essential to train experts, create comprehensive guidelines, implement quality controls, and refine annotations iteratively. Addressing ambiguity and ensuring consistent, reliable annotations are key.
Applications and Ethical Considerations
Medical image annotation has wide-reaching implications for disease diagnosis, treatment planning, medical education, and AI training. Ethically, it demands attention to data privacy, bias prevention, and regulatory compliance to maintain patient trust and data integrity.
Looking Ahead
The future of medical image annotation lies in AI advancements, multi-modal data integration, privacy-preserving techniques like federated learning, and standardized practices. These trends promise to further enhance healthcare outcomes and research while meeting ethical standards.
Conclusion
In the dynamic landscape of healthcare, the fusion of AI data annotation, machine learning, and medical data annotation in healthcare has emerged as a game-changer in disease detection. The meticulous process of annotating medical images paves the way for developing powerful algorithms capable of identifying and diagnosing diseases with unprecedented accuracy. As technology advances, the role of medical annotation in disease detection is poised to become even more pivotal, shaping the future of healthcare and saving lives through early detection and intervention.