Managing Large-Scale Annotation Projects
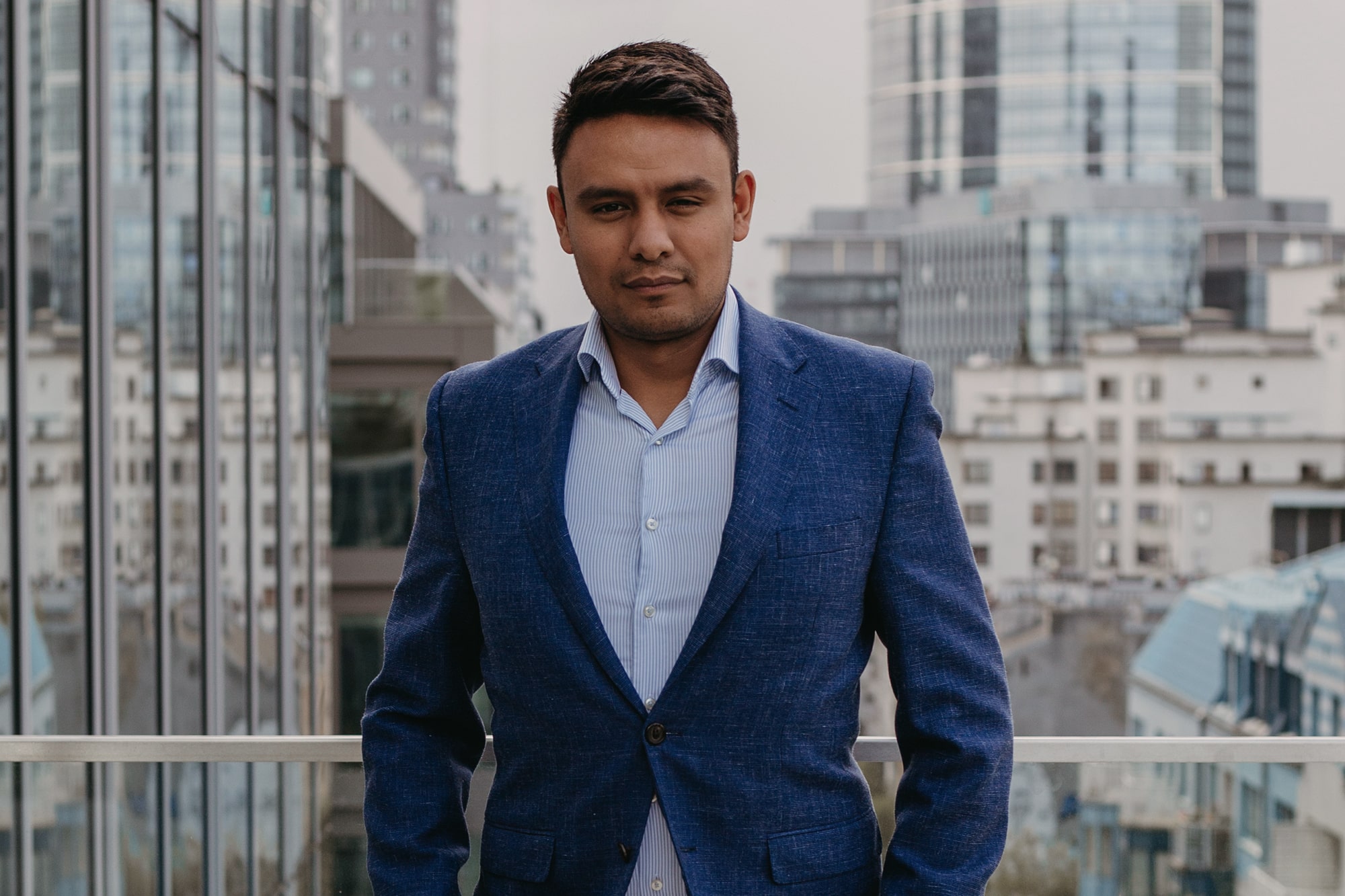
In the rapidly evolving landscape of artificial intelligence, high-quality annotated data serves as the cornerstone for training robust machine learning models. As AI applications become more sophisticated and diverse, the demand for large-scale annotation projects is on the rise. However, managing such projects comes with its own set of challenges. In this article, we delve into effective strategies for managing large-scale annotation projects, ensuring accuracy, efficiency, and timely delivery.

The Challenge of Scale
Large-scale annotation projects involve annotating vast amounts of data, often across multiple domains and modalities. This can encompass images, text, audio, video, and more. The complexity of managing these projects demands a systematic approach to ensure that the labeled data is of high quality and aligned with the project's goals.
The Benefits of Effective Management
Managing large-scale annotation projects is no small feat, but the rewards are significant:
- High-Quality Data: Effective management ensures that the labeled data is accurate, consistent, and meets the project's requirements.
- Efficiency: Well-defined workflows and task allocation optimize the use of resources, saving time and effort.
- Timely Delivery: Clear milestones and tracking mechanisms help meet deadlines and deliver results on time.
- Client Satisfaction: Transparent communication and regular updates build trust with clients, enhancing their satisfaction.
- Learning and Improvement: Feedback loops enable continuous improvement in annotation quality and processes.
Conclusion
Large-scale annotation projects are pivotal for training AI models that can revolutionize industries. By implementing thoughtful strategies for project management, organizations can navigate the challenges of scale while maintaining data quality, efficiency, and client satisfaction. As the AI landscape continues to evolve, effective management will remain a cornerstone of success in data annotation.