NLP's Role in Conversational AI

Recent advancements in natural language processing (NLP) and conversational AI are transforming our interactions with machines and each other. These technologies, rooted in machine learning and AI, enable machines to understand, interpret, and generate human language. Historically, human communication has evolved from primitive pictograms to complex language systems. The advent of AI systems like chatbots that understand natural language has marked a significant leap in linguistic communication.
Conversational AI, increasingly prevalent, facilitates interaction with machines using natural language, either spoken or written. NLP, a subset of AI focusing on human language processing, is critical to this technology. This article delves into the role of NLP in shaping conversational AI and the key components driving its effectiveness.
Conversational AI encompasses chatbots, virtual assistants, and voice-activated devices, all designed to simulate human-like conversations. Its aim is to enable effortless and natural user interactions, bypassing conventional input methods like keyboards or touchscreens.
Statistics highlight conversational AI's impact: About 90% of businesses using these tools report faster handling of calls and complaints, per MIT. In the US, 43% of digital banking users prefer live chats or bots for problem resolution. In the HR sector, 35% of companies in 2022 adopted conversational user experiences and NLP for recruitment processes.
While the market for Conversational AI is expanding, NLP remains a critical underpinning. The global NLP market is projected to reach $63.37 billion by 2030, with key sectors including language translation, speech-based, and text-based NLP.
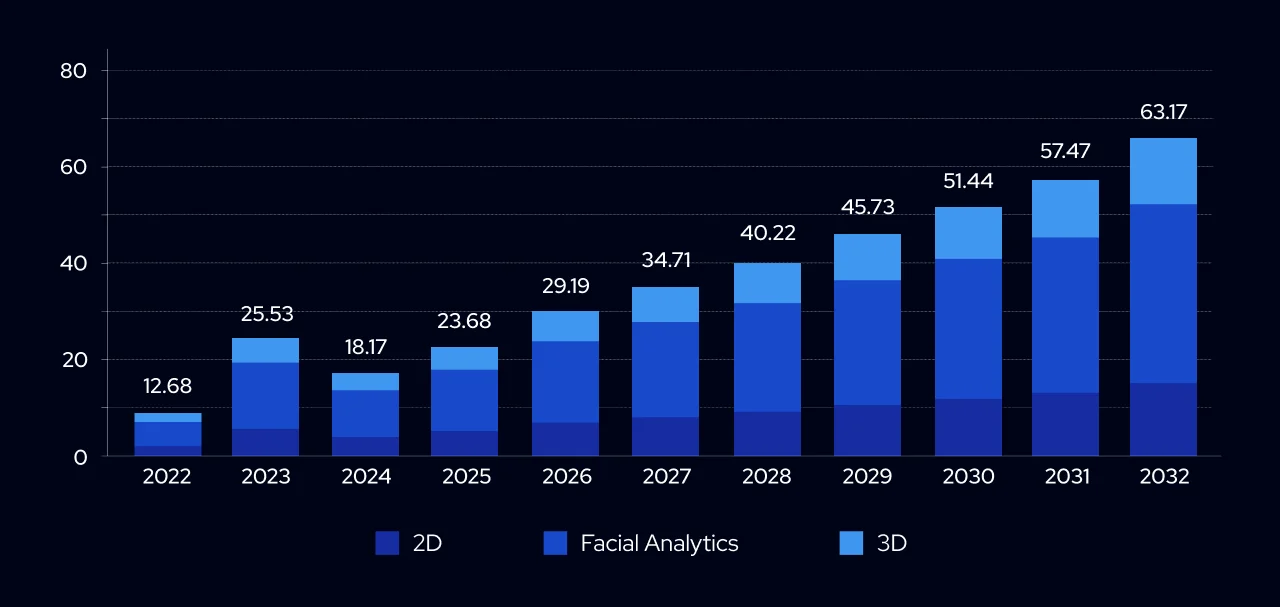
The Importance of NLP in Enhancing Conversational AI
Understanding the Significance of NLP
1. Handling Extensive Textual Data
Natural Language Processing (NLP) is pivotal in enabling computers to interact with humans in their own language, thus facilitating the scaling of language-related tasks. NLP empowers computers to read and understand text, listen to and interpret speech, assess sentiments, and identify key elements in communication. In today's world, machines can analyze language data more efficiently and consistently than humans. Given the enormous volume of unstructured data generated daily, ranging from social media posts to medical records, automation through NLP is essential for effective and comprehensive analysis of textual and speech data.
2. Organizing Diverse and Complex Language Data
Human language is notably varied and intricate. Our expressions, whether verbal or written, can take countless forms across numerous languages and dialects, each with its own vocabulary, grammar, and colloquialisms. Written communication often includes abbreviations and omits punctuation, while spoken language is characterized by regional accents, mumbling, stuttering, and the incorporation of foreign words. While current approaches like supervised and deep learning attempt to model human language, they often fall short in incorporating domain knowledge or understanding the syntactic and semantic structures. NLP stands out for its ability to convert language into valuable numeric data and address ambiguities in language.
NLP's role in the evolution of conversational AI cannot be overstated. It enables machines to understand, interpret, and generate human language, making it possible to process and analyze the vast quantities of textual data that are inherent in human-machine communication.
The integration of Natural Language Processing (NLP) into conversational AI is pivotal, encompassing various components and processes that enhance the interaction between humans and machines. Key aspects include:
- Speech Understanding and Recognition: Conversational AI systems leverage NLP's Automatic Speech Recognition (ASR) to convert spoken words into text, enabling real-time understanding of voice inputs.
- Sentiment Analysis: NLP allows the detection of sentiments in text - positive, negative, or neutral. This enables AI systems to tailor responses based on the emotional tone of the user, enhancing overall experience.
- Entity Extraction and Intent Recognition: NLP techniques help machines grasp user intentions by extracting entities and key elements, ensuring more accurate and context-aware responses.
- Machine Translation: Enhanced by NLP, machine translation facilitates communication between users of different languages, crucial for multilingual conversational AI.
- Language Understanding and Semantic Parsing: NLP aids in comprehending sentence structures and meanings, allowing AI systems to handle complex queries and provide precise answers.
- Contextual Understanding and Dialogue Management: NLP enhances AI's ability to maintain context throughout conversations, leading to more personalized and insightful responses.
- Text Generation and Summarization: Language modeling and text generation algorithms enable machines to produce coherent, context-relevant dialogues.
- Named Entity Recognition (NER): NLP assists in identifying and categorizing named entities in text, such as names and places, enhancing the AI's understanding of specific user query details.
Additional NLP components crucial for conversational AI:
- Tokenization: Breaking down text into tokens (words, phrases) prepares it for further analysis, aiding the AI in sentence structure understanding.
- Part-of-Speech (POS) Tagging: Identifying each token's grammatical role improves AI's capability in generating grammatically correct responses.
- Dependency Parsing: By identifying syntactic relationships, this helps the AI comprehend sentence meanings and produce relevant responses.
- Semantic Role Labeling (SRL): This involves pinpointing the roles within sentences, such as the agent and action, aiding in generating appropriate responses.
- Language Modeling: Essential for creating human-like responses, these models predict word sequences, ensuring coherence and relevancy in AI-generated text.
The evolution of conversational AI and NLP, transitioning from basic rule-based frameworks to sophisticated deep learning models, represents a remarkable leap in technology. This transformation has revolutionized our interaction with machines, fostering a more natural and instinctive communication experience. Looking ahead, the prospects for further advancement in personalized AI conversations are limitless, heralding an era where machines can genuinely comprehend and aid us in ways that closely resemble human interaction. Yet, it's crucial to navigate this future thoughtfully, taking into account ethical considerations and striving to ensure that these technological advancements serve the broader good of humanity.